Leveraging Machine Learning for Enhanced Mental Health Diagnoses
Written on
Chapter 1: Understanding the Role of Machine Learning
In my background in research, I have developed a passion for translating complex academic findings into accessible language. This helps stimulate my thinking while keeping the public informed. Recent studies from the University of Birmingham and PRONIA have revealed that machine learning can be instrumental in accurately identifying symptoms related to psychosis and depression.
It’s crucial to note that individuals with depression often present symptoms that overlap with those of psychosis. As a result, a clinician may assign a primary diagnosis of one condition while listing the other as secondary. For instance, if a patient is primarily diagnosed with psychosis, depression may be recorded as a secondary issue. Consequently, treatment typically emphasizes the psychotic symptoms such as hallucinations and delusions.
Determining the accuracy of these diagnoses is challenging, especially when taking into account a person’s unique personality traits, social contexts, and intricate neurobiological factors.
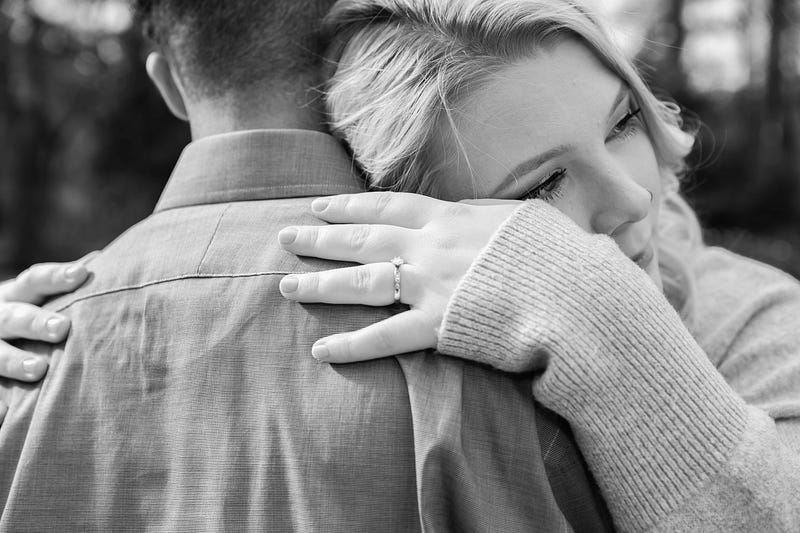
Photo by Moe Kong on Unsplash
Chapter 2: The Intervention of Machine Learning
Here’s where the application of machine learning becomes significant. The researchers aimed to develop a precise model for diagnosing psychosis and depression, thereby enhancing diagnostic accuracy.
Initially, they studied 300 patients exhibiting symptoms of both conditions. Because these symptoms were equally pronounced, establishing a single primary diagnosis proved difficult. The existing diagnostic framework tends to favor one condition over the other, forcing clinicians to label one as primary and the other as secondary.
The researchers conducted thorough assessments, including detailed questionnaires, clinical interviews, and data from structural magnetic resonance imaging (MRI). This allowed them to isolate cases of “pure” depression and “pure” psychosis. Subsequently, machine learning techniques were applied to patients who displayed symptoms of both conditions.
The findings indicated that individuals identified with depression as the primary condition received more accurate diagnoses compared to those without this designation. This suggests that depression plays a more significant and interconnected role than psychosis. Furthermore, it can be argued that machine learning algorithms have the potential to simplify the complexities surrounding these two mental health disorders, for better or worse.
As noted by Pedro Domingos,
“[T]he greatest benefit of machine learning may ultimately be not what the machines learn but what we learn by teaching them.”
The first video, "How Do We Make Human-Centered AI for Mental Health Prediction in Social Media Data?", explores the integration of AI with mental health assessments, emphasizing the need for a human-centered approach.
The second video, "CNStalks: Using machine learning to predict mental health - Andre Marquand," discusses the application of machine learning techniques in predicting mental health outcomes, shedding light on innovative methodologies in the field.
For more articles from the author, please read:
- Explaining Countertransference in Healthcare
- Thoughts From an Aspiring Therapist
- The Psychology Behind Baby-Talk