Exploring Reinforcement Learning: On-Policy vs Off-Policy Dynamics
Written on
Understanding the Concepts of Reinforcement Learning
In this section, we simplify the concepts of On-Policy and Off-Policy in reinforcement learning for clarity.
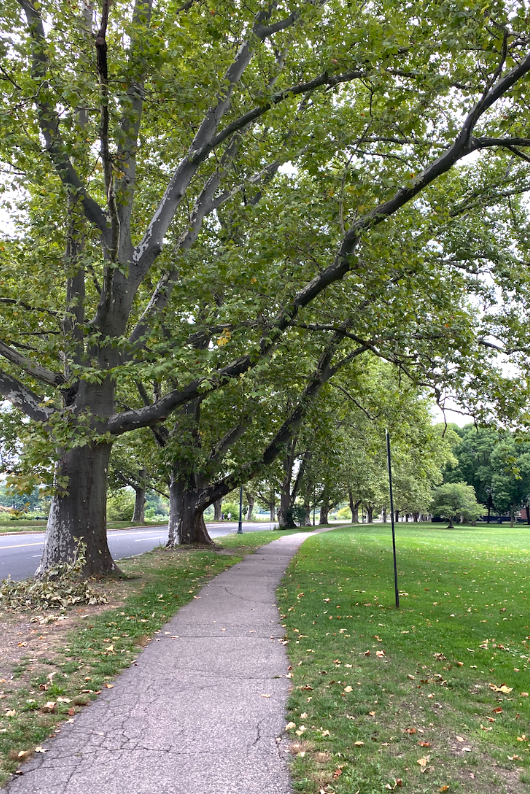
Imagine you've recently relocated and have sampled various eateries in your neighborhood. Today, you're set to dine out once more. We can frame the challenge of identifying the ideal restaurant as a reinforcement learning scenario.
You, the Agent, are on a quest to discover the finest dining experience in your area, which we refer to as the Environment. Each time you visit a restaurant, you interact with the environment, which alters your state—essentially your dining experience. Depending on where you eat, you receive a numerical reward that reflects the quality of your dining experience. Your primary goal as the Agent is to maximize your cumulative reward, ultimately leading to the best restaurant experience over time.
The Role of Policy in Decision Making
A policy serves as a guideline for an agent in pursuit of its objectives.
A policy determines the actions an agent will take within an environment to optimize its long-term rewards. The optimal policy is one where the expected reward meets or exceeds that of any other policy across all states. In your quest for the best dining experience, the choices you make form your policy or strategy.
The policy an agent employs evolves based on its experiences while navigating the environment. You may opt for your go-to restaurant based on prior experiences, thereby exploiting the best-known information. Conversely, you might decide to explore a new dining option, which could lead to an exceptional experience or an average one.
This scenario exemplifies the Exploration-Exploitation dilemma—choosing between leveraging known information (exploitation) and exploring new possibilities (exploration).
Exploration involves the agent enhancing its knowledge about possible actions that might yield long-term benefits, while exploitation focuses on utilizing existing knowledge to maximize immediate rewards. Striking a balance between these two approaches is crucial, as an agent needs to sample various actions to truly understand the environment and enhance long-term rewards.
When an agent leans towards exploitation, it employs a greedy policy, opting for actions that promise the highest known value. However, if the current value isn't optimal, the greedy policy itself may not yield the best outcomes.
To manage the balance between exploration and exploitation, the epsilon-greedy method comes into play, allowing the agent to randomly choose between exploring new options and exploiting known ones.
In the video "Reinforcement Learning: on-policy vs off-policy algorithms," the differences between these two types of learning strategies are discussed in detail.
On-Policy vs. Off-Policy Learning
On-policy methods focus on evaluating or enhancing the same policy used for decision-making. This can be likened to your personal exploration of restaurants, where you assess and improve your choices based on firsthand experience.
On the other hand, off-policy methods utilize a behavioral policy for exploration while collecting samples to inform a second, optimized target policy.
The behavioral policy dictates the actions taken by the agent, while the target policy learns from the rewards obtained and updates the Q-values accordingly.
When the target and behavioral policies differ, the agent is identified as an off-policy learner. Conversely, if both policies align, the agent is recognized as an on-policy learner.
An illustrative example of off-policy learning would be using Google Maps to find the best restaurants. Here, the recommendations serve as the behavioral policy, while your decision to follow or disregard those suggestions represents the target policy.
The video "Off Policy vs On Policy Agent Learner - Reinforcement Learning - Machine Learning" further elaborates on these concepts and their applications.
Importance Sampling in Policy Optimization
Importance sampling is a technique used to predict the probability of rare events. It is particularly valuable in off-policy methods, allowing for variance reduction and improved estimates through weighted sampling.
When a new, highly-rated restaurant opens nearby, but few have experienced it, importance sampling can help gather data from this significant yet under-visited option. By weighting returns according to the importance-sampling ratio, agents can gain insights that would otherwise be missed.
Summary
Reinforcement learning revolves around finding an optimal policy, with agents grappling with the exploration-exploitation dilemma. By leveraging a greedy policy, agents exploit existing knowledge for optimal rewards. The epsilon-greedy approach seeks to harmonize exploration and exploitation. To identify an optimal policy, simultaneous policy improvement and evaluation are essential. On-policy and off-policy methods present distinct strategies for achieving this goal. The former employs the same policy for evaluation and enhancement, while the latter uses a behavioral policy for exploration alongside a target policy for improvement.
References
Reinforcement Learning: An Introduction by Richard S. Sutton and Andrew G. Barto